AI generated font projects to inspire you
AI tools are slowly making their way into type design, and I'd like to highlight a few projects that inspired me in using AI-generated fonts.
Machine Learning Font
Before starting my individual experiments, I will first describe some references in which AI tools were used in type design. These projects have inspired me and at the same time challenged me to break new ground.
The Machine Learning Font project was published by NaN studio at the end of 2019. A styleGAN model, which was state of the art at the time, was trained via RunwayML. The training data was processed from 2,674 Google fonts by a program into individual image-per-glyph images. Due to the automation of this process, the training data was not selected completely accurately and the generated results often show a bleed.
It is interesting to see that even with 10,000 training steps very realistic letters were generated. The only value that can be changed in this model is the truncation. It indicates how diverse the results will be: The lower the value, the more similar the letters are generated.
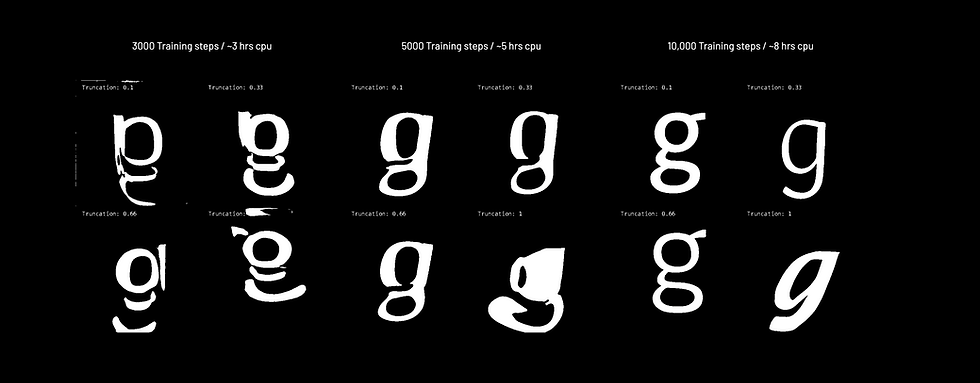
AlphabetGAN
Runway also provides very low-threshold access to a model that has already been trained with 372,450 characters. This is called AlphabetGAN and was created by Sujan Dutta. In the preliminary stages of my experiments, I tried this one out. Here you can also see the influence of the truncation value on the variance of the results.

Generating all letters of the Latin alphabet at once has the advantage of generating a consistent font, but the resolution of a single letter is 28x28 pixels. This makes it difficult to continue working.
Bit Tripper
The Bit Tripper project by Counterpoint and YACHT is an interactive tool for exploring generative typography powered by deep neural networks. A GAN model was trained with a data set of bitmap fonts to generate fonts from the latent space of the model. Visitors to the website of the same name can download these fonts as OpenType fonts. What is interesting for me here is the focus on one font category in the data set.
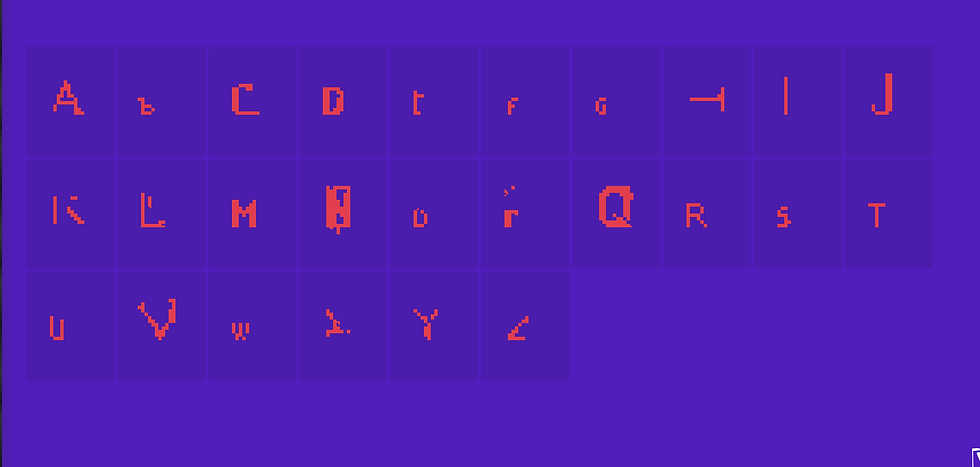
From these projects I have learnt that I want to be extra careful when creating the training data. I will not work with GANs, but try out the new technology of diffusion models in the field of type design. Since my generated images should be as high-resolution as possible, I do not aim to generate a complete Latin alphabet and focus on individual letters and their properties. I want to find out how different font categories in the training data affect the model and how these categories can be generated.
コメント